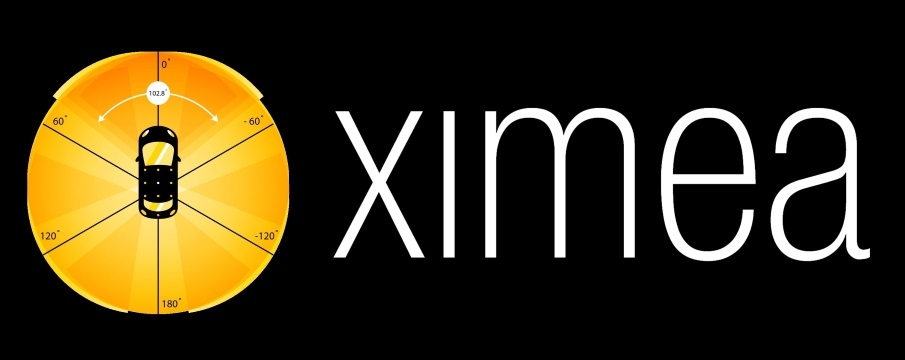
- Home
- Discover
- Your applications
- Case studies
- Case study: How XIMEA cameras power Driverless cars
Autonomoose Self-driving cars
What seems a distant future for many, is already a reality for the chosen few: “Autonomous Driving” represents one of the hottest topics in the research fields like Natural science and Engineering.
Thanks to the combination of these two fields and the collaboration between the University of Waterloo and the University of Toronto, autonomous driving has an identity now, Autonomoose.
Autonomoose represents the one-of-a-kind self-driving car fitted with high performance cameras from XIMEA that provide “the eyes” to the vehicle.
Moreover, the synchronization of the radar scanners, sonar, lidar, vision sensors, and the sophisticated on-board computer with a self-driving code base make the driving suitable even for difficult winter weather conditions.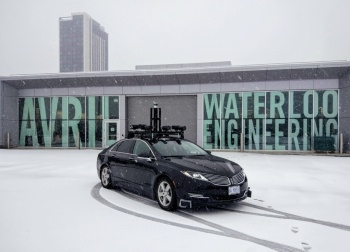
What is Autonomoose?
The researchers from the University of Waterloo Centre for Automotive Research (WatCAR) are modifying a Lincoln MKZ Hybrid to autonomous drive-by-wire operation.
They are working on a research platform named ‘Autonomoose’ and here are its contents:
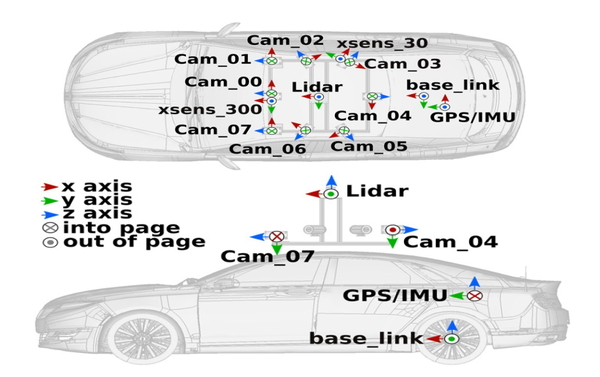
- A full suite of radar, sonar, LIDAR, inertial and vision sensors.
- Powerful embedded computers to run a completely autonomous driving system, integrating sensor fusion, path planning, and motion control software.
- Custom autonomy software stack being developed at Waterloo as part of the research.
Based on the SAE International standard for automated driving (SAE J3016, a 0-5 scale), the vehicle will initially operate at level 2.
Through the duration of the research program, the team will advance the automation to level 3 and ultimately level 4, which has been reached in 2018.
Who is taking part in the project?
The two main universities who are participating in the project: the University of Toronto and the University of Waterloo.
Founded in 1827, the University of Toronto has evolved into Canada’s leading institution of learning, discovery and knowledge creation.
The ideas, innovations and actions of more than 560,000 graduates continue to have a positive impact on the world.
In just half a century, the University of Waterloo, located at the heart of Canada’s technology hub, has become a globally focused institution, celebrated as Canada’s most innovative university for 23 consecutive years.
Waterloo is home to the world’s largest post-secondary cooperative education program and encourages enterprising partnerships in learning, research and discovery.
In the next decade, the university is committed to building a better future for Canada and the world by championing innovation and collaboration to create solutions relevant to the needs of today and tomorrow.
Altogether, nine professors are involved from the Faculty of Engineering and Faculty of Mathematics coming from the two universities.
Additionally, the Natural Sciences and Engineering Research Council (NSERC) provided initial research funding.

How does autonomous driving work in winter?
A Lincoln MKZ hybrid carrying a full sensor suite roves the snowy roads of Ontario, recording bad-weather data to empower driverless vehicles that will eventually brave these conditions.
Driving algorithms generated by data collected in good weather can become confused, and miscalculate badly, because of different visual conditions in snowy weather with extensive white backgrounds.
Some points from the Feature set of Autonomoose:
- GPS trajectory tracking - high precision, dual antenna GPS/INS used to follow cubic spline path with bounded curvature; simple blended velocity profile control; speed profile defined to maintain traction; nonlinear path tracking controller
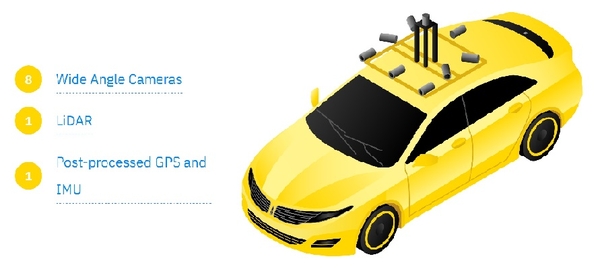
- Obstacle and vehicle detection - Computation constrained solution: LIDAR ground plane removal; LIDAR occupancy grid mapping; hypothesis generation from Radar, V2V; Target clustering; Bounding box alignment and tracking
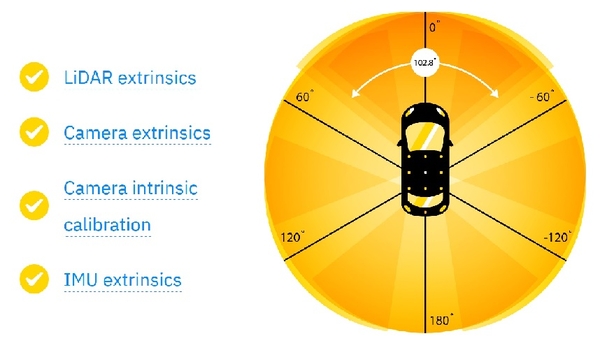
- Local motion planning - Multi stage approach: Optimal static path planning; Fixed and state sampling; Static collision checking; Velocity profile generation, Extremely fast: 1-2 ms per path on ARM57 core; 10-20 ms for a complete solution
What is inside Autonomoose?
The Autonomoose’s suite of synchronized sensors includes:
- 8 cameras - MQ013CG-E2 from XIMEA,
- LiDAR scanner - Velodyne VLP-32C,
- GPS/inertial system - Novatel OEM638, incorporating a Sensonor STIM300 MEMS IMU,
- 2 additional IMUs - (Xsens MTi-300- and 30-AHRS) as part of the advanced driver assistance system (ADAS)
The suite records and timestamps 10 images per second.
The LiDAR is time-synchronized with the GPS PPS signal and NMEA messages.
Each LiDAR point cloud contains a full 360° sweep of the LiDAR beams, starting from the 180° cut angle directly behind the car and rotating clockwise.
The Autonomoose computer receives the GPS PPS signals and NMEA messages.
A Novatel Inertial Position Velocity Attitude-Extended (INSPVAX) message contains the most recent position, velocity and orientation as well as standard deviations.
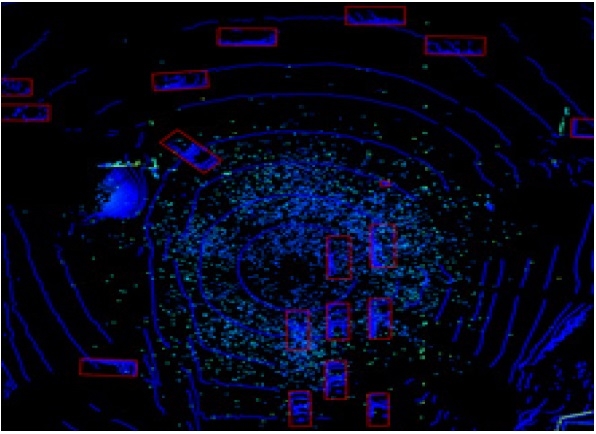
Why is data important in autonomous driving?
Steven Waslander, an associate professor at the University of Toronto Institute for Aerospace Studies in the Faculty of Applied Science & Engineering, and Krzysztof Czarnecki, a professor at the University of Waterloo, lead the team that is compiling the Canadian Adverse Driving Conditions (CADC) dataset.
A collaboration with Scale AI helps them to categorize the data.
“Data is a critical bottleneck in current machine-learning research,” said Alexandr Wang, Scale AI CEO. “Without reliable, high-quality data that captures the reality of driving in winter, it simply won’t be possible to build self-driving systems that work safely in these environments.”
The project has placed the data, documentation and support tools in GitHub.
An open-access scientific article on arXiv explains the data collection process.
The dataset contains 7,000 frames collected through a variety of winter driving conditions.
LiDAR frame annotations that represent the ground truth for 3D object detection and tracking have been provided by Scale AI.
“Bad weather is a condition that is going to happen,” said Waslander. “We don’t want Canada to be 10 or 15 years behind simply because conditions can be a bit tougher up here.”
Conclusion
The future of transportation is taking a new shape.
Thanks to the collaboration between two of the leading universities across Canada, autonomous vehicles, in particular Autonomoose, are setting high standards and leading to higher goals, especially in regard to the SEA levels.
The shift from conditional automation to high automation is other than easy and requires automated driving systems that monitor the driving environment and are capable of operating in compliance with the district´s applicable traffic laws.
To reach this automation level, visual sensors are the key to understanding the driving environment, as is the case in Autonomoose with XIMEA´s eight MQ013CG-E2 cameras equally distributed across the vehicle.
On the one hand, the idea is very simple - outfit a car that can autonomously decide when to steer.
On the other hand, the implementation elides a lot of complexity.
That is why the top Canadian Universities are taking outstanding researchers to push the boundaries of autonomous driving to the limit.
The ground-breaking work behind Autonomoose is not only contributing to the field of autonomous driving in enormous ways, but is also reshaping our future society.
Contacts and Links for Autonomoose
The following professors lead the research team:
Steve Waslander, Mechanical and Mechatronics Engineering
Krzysztof Czarnecki, Electrical and Computer Engineering (WISE lab)
Press contact:
Ross McKenzie
Managing Director, WatCAR
Waterloo Centre for Automotive Research
University of Waterloo
E-mail: ross.mckenzie@uwaterloo.ca
Website: https://www.autonomoose.net/
University of Waterloo: cadcd.uwaterloo.ca
Wave Laboratory: http://wavelab.uwaterloo.ca/
Canadian Adverse Driving Conditions Dataset: https://arxiv.org/abs/2001.10117
Pictures and video Courtesy U. Toronto and U. Waterloo Canadian Adverse Driving Conditions project.
Related articles
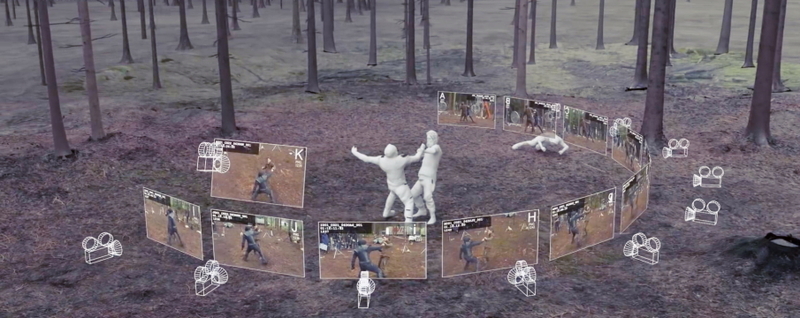
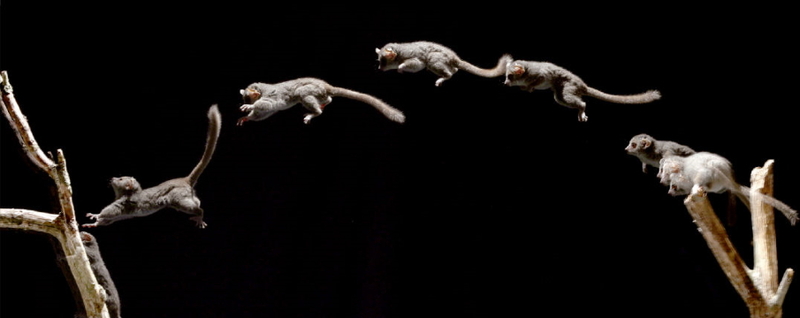
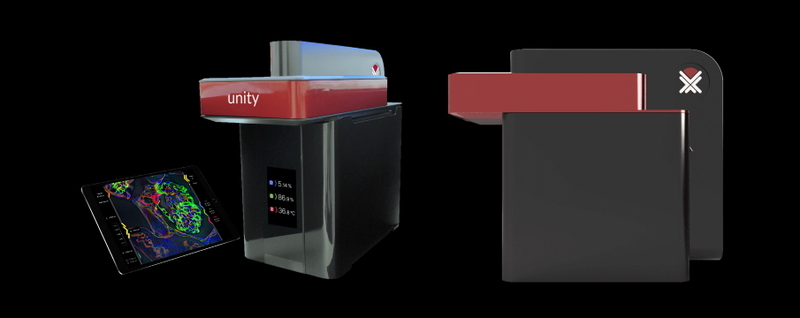
Latest articles
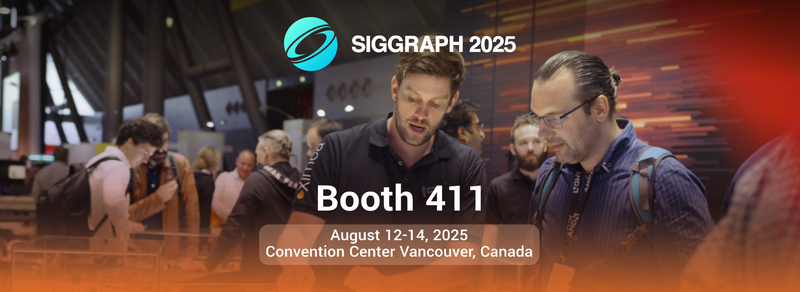
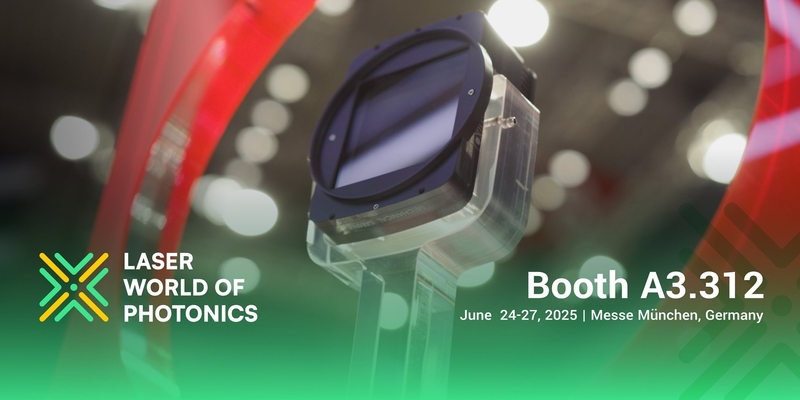
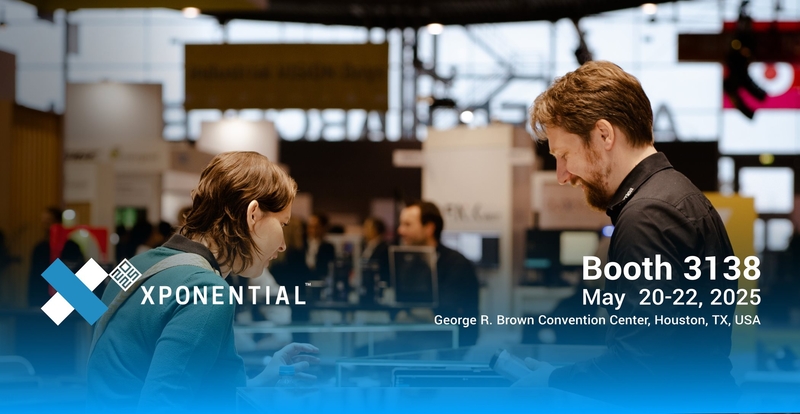
Join us at booth #3138!
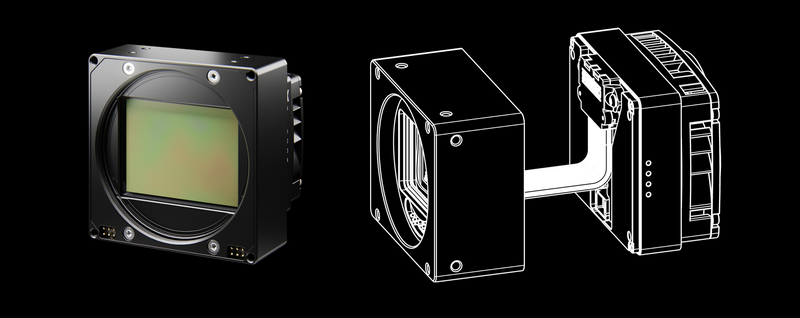
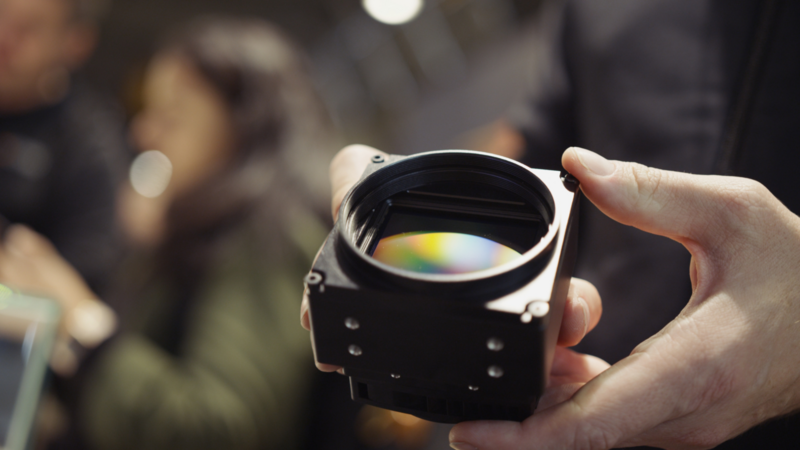
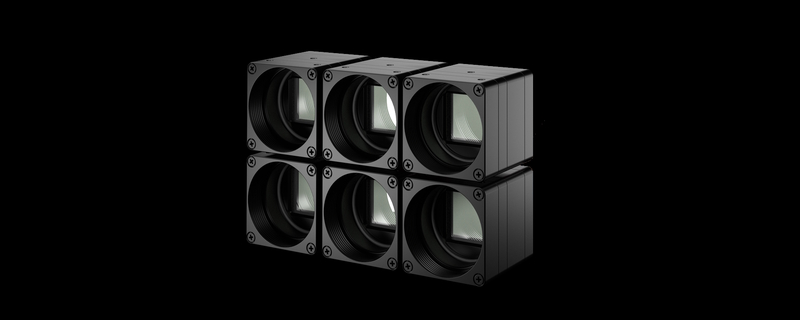